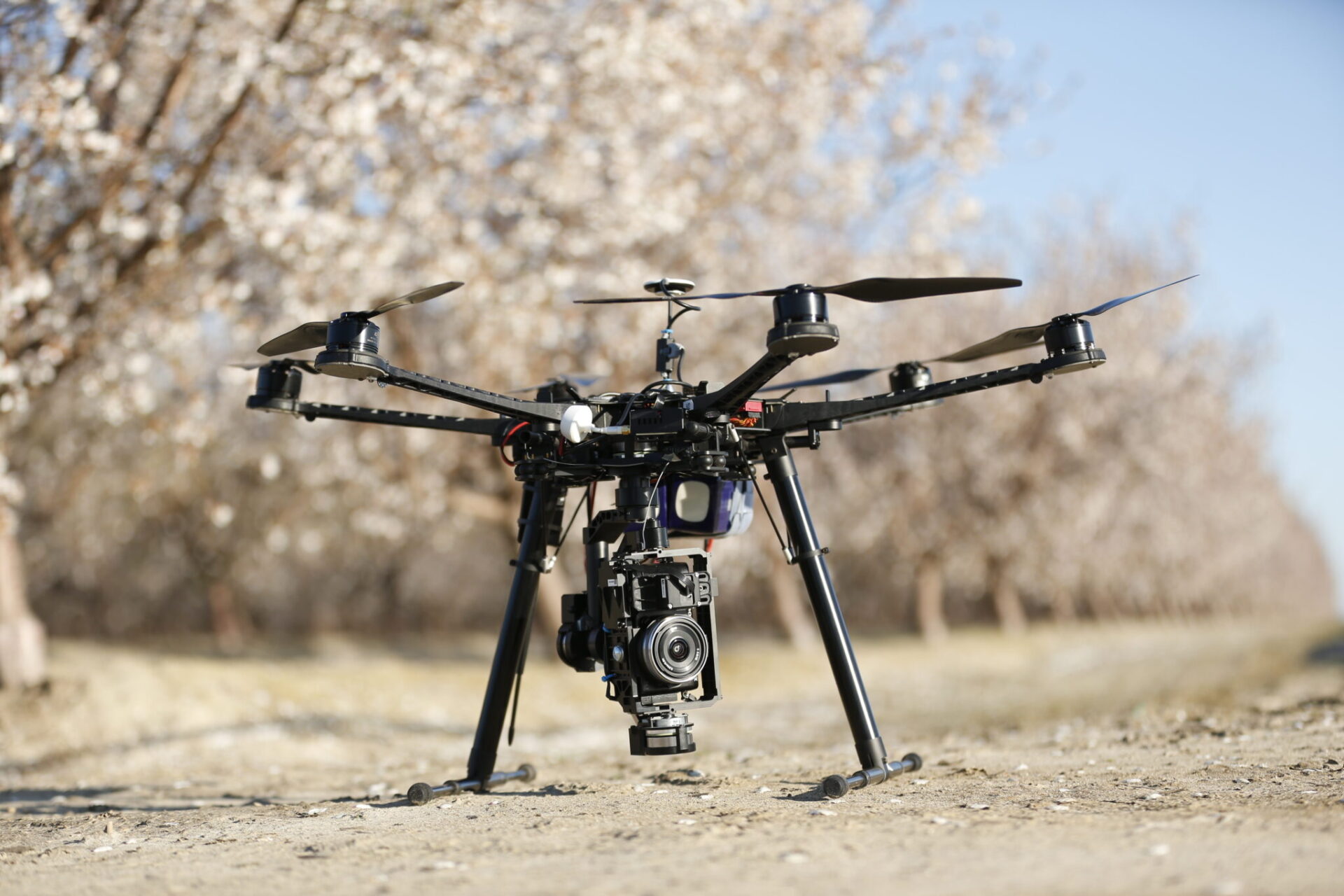
Unmanned Aircraft Systems (UAS) are increasingly being utilized in farming applications throughout California’s Central Valley. The Federal Aviation Administration (FAA) anticipates that the adoption of UAS technology will continue to dramatically increase over the next several years.
In 2019, there were 900,000 registered UAS drones in the United States, with about 17% being utilized for agriculture, meaning there are more than 153,000 drones currently in use for farming applications. As California farms produce roughly 13% of US farm dollars in the United States, it can be estimated that there are about 20,000 agriculture drones in California today. Additionally, the 2017 census noted that there were 124,405 farmers and 70,521 principle operators in California, meaning roughly 1 in 10 California farmers and 1 in 5 principle farm operators currently utilize UAS technology as part of their farming operations. It is important to note that these estimates have doubled over the past couple of years and, if the trend continues, it is anticipated that the majority of farmers will utilize drone technology to some degree over the next decade.
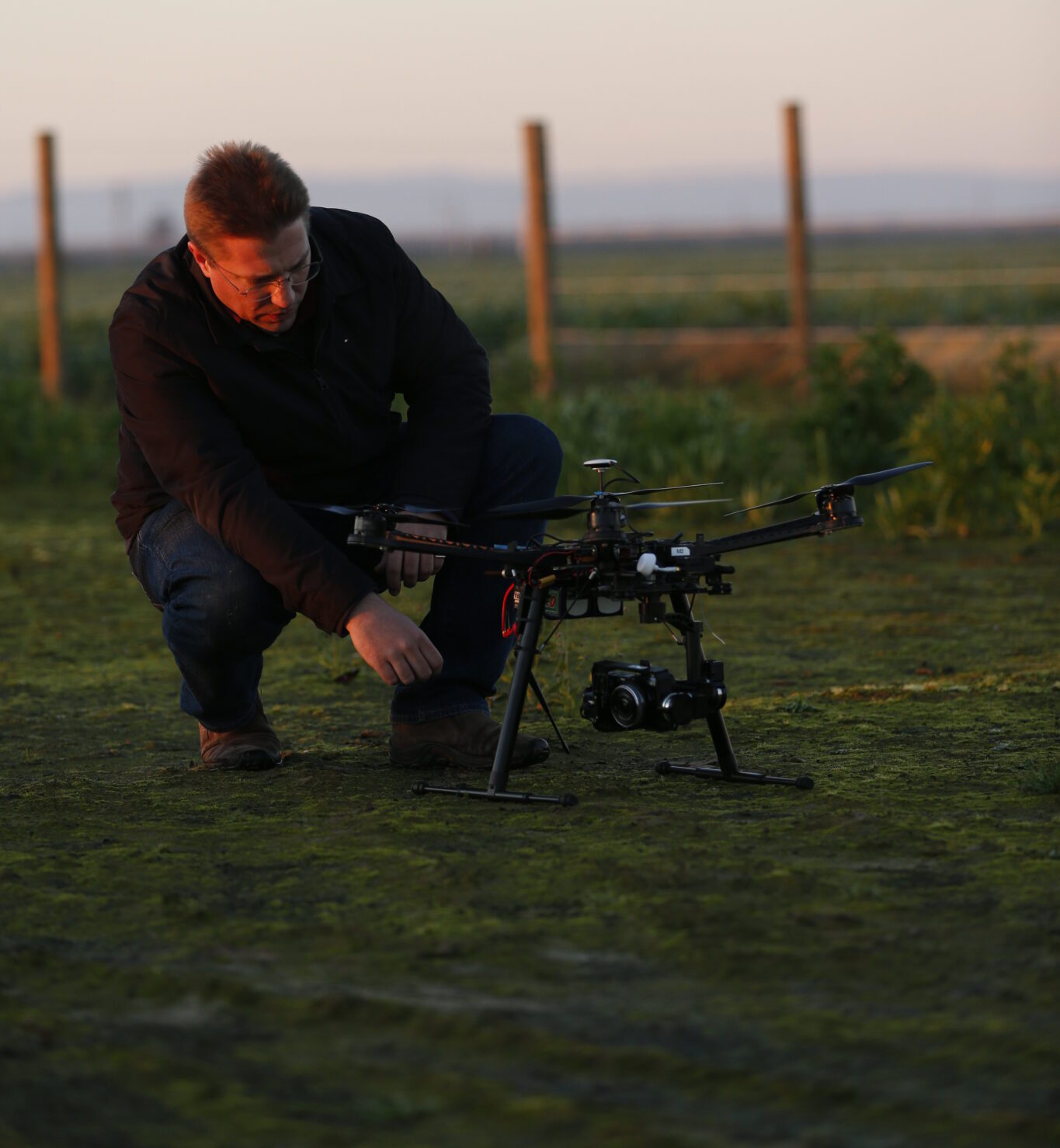
Integrated Technology
Although aerial imaging has been previously used within agriculture for over 50 years to monitor crop conditions (including pest, weed, disease monitoring, damage observations due to drought and flooding, and growth differences due to soil chemical and physical problems,) it is the integration of imaging systems with global positioning systems (GPS), recent advancements in UAS autonomous flight capabilities, and the miniaturization of flight technology enhanced with micro-electromechanical systems (MEMS) that has allowed the UAS industry to take off.
Over time, the focus has shifted from the development of basic flight technologies (electrical motors, battery technology, GPS, magnetometers, gyroscopes, accelerometers, etc.), to low-cost imagers and multi-spectral devices for imaging and photogrammetry, toward autonomous flight and automated data collection, and finally, to integrated systems that have the potential to provide actionable intelligence and prescription maps to the farming community.
Most imaging capabilities utilized by drones currently include taking photographs measuring the reflectance off of a canopy in the Red, Green and Blue (RGB) color bands (400–700 nm) to produce visible RGB images, in addition to near-infrared (NIR) wavelengths (800–850 nm) where strong scattering starts to occur due to the leaf’s internal structure, and the so-called red edge spectral bands (the wavelengths between 700–800 nm, where chlorophyll absorption stops.) The reflectance values within each color band are normalized between 0 (no reflectance) and 1 (complete reflectance), and are combined using sums and differences to produce vegetation indices that attempt to correlate the spectral information against the Crop Water Stress Index (CWSI) to determine how much stress a plant or tree is currently experiencing.
A variety of vegetation indices have been developed over the years, including the Normalized Difference Vegetation Index (NDVI), Green NDVI and Normalized Difference Red Edge Index (NDRE), all of which examine the degree to which chlorophyll absorption is occurring within the visible spectrum and compare the result against expected higher reflectance in the NIR or Red Edge (RE) band(s). In a healthy canopy, there is a strong difference between the visible and infrared reflectance (producing an index closer to 1.) When a plant or tree experiences stress, the indices generally trend closer toward 0.
Additionally, when many pictures are taken over a large field, sophisticated digital imaging software can then “stitch” the images together to produce an orthomosaic map in which the aerial photograph is geometrically corrected (orthorectified) to keep the scale uniform across the entire set of images as they are stitched together. The goal is to produce a high-resolution vegetative image over a much larger area that has been corrected for camera tilt, lens distortion and topographic relief so that true distances can be measured and accurate decisions be made within the field.
Remote Sensing
When properly used, the integration of UAS technology with remote sensing and imaging has the potential to readily produce prescription maps that can then be uploaded to variable rate spreaders capable of using real-time GPS sensor information for precision agriculture. A recent case study by AeroVironment demonstrated that a walnut grower in Modesto, Calif. was able to increase his yields by 21% in a 40-acre field by altering irrigation and nitrogen applications in the northern part of his field based upon the NDVI images that were taken over a two-year period using drone imaging.
However, there are several other things to keep in mind when considering this technology:
- Accurate NDVI, NDRE or other vegetative indices are highly dependent upon obtaining accurate reflectance values. Since reflectance measurements can change significantly over the course of the day and under different weather conditions and seasons, it is important to operate such imagers under the same conditions each time data is collected. This is especially important for tree-based crops (i.e. almonds or walnuts), since tree canopies are much more variable and more loosely correlated against stem-water potential and water stress when using remote imaging.
- Vegetative indices produce relative measurements that must be properly calibrated to produce actionable data. In years past, a calibration target had to be placed in the field so that the images could be calibrated against the known reflectance of the target pad. Today, most imagers should contain a secondary sensor that points toward the sun (such as the MicaSense RedEdge) that serves to properly calibrate the measured reflectance values. Regardless, the imaging device must have its reflectance values properly calibrated every time it is utilized to produce accurate data.
- Orthomosaic vegetative maps still need to be calibrated against “boots on the ground” to provide a baseline understanding of what is happening within the field. The vegetative index maps that are created by these imagers are still relative – it is up to the farmer to determine at what values between 0 and 1 is true stress occurring. Toward that end, differential NDVI or NDRE maps are recommended, in which multiple sets of vegetative maps are created over several days and/or weeks so that changing trends within the field can be determined. Several case studies have demonstrated farmers have been able to identify localized stress (such as disease) down to the tree level in almond orchards when using differential NDVI and NDRE images.
Additionally, UAS technology provides more tools for precision agriculture than just imaging. Within areas of rough terrain, drones have been used to collect data from remote sensors. This technology can readily be applied within large agricultural fields, reducing the need for transmitters, repeaters, and the associated wiring and infrastructure.
Wireless sensors and sensor networks have evolved from requiring custom manufacturing limited by large power consumption and short duration (hours to days,) heavy weight (kilograms) and large footprints to commercial companies providing embedded solutions that can be powered by standard batteries (AA, 9V, etc.) with significantly reduced weight (grams or lower) and size (tens of centimeters down to sub millimeters.)
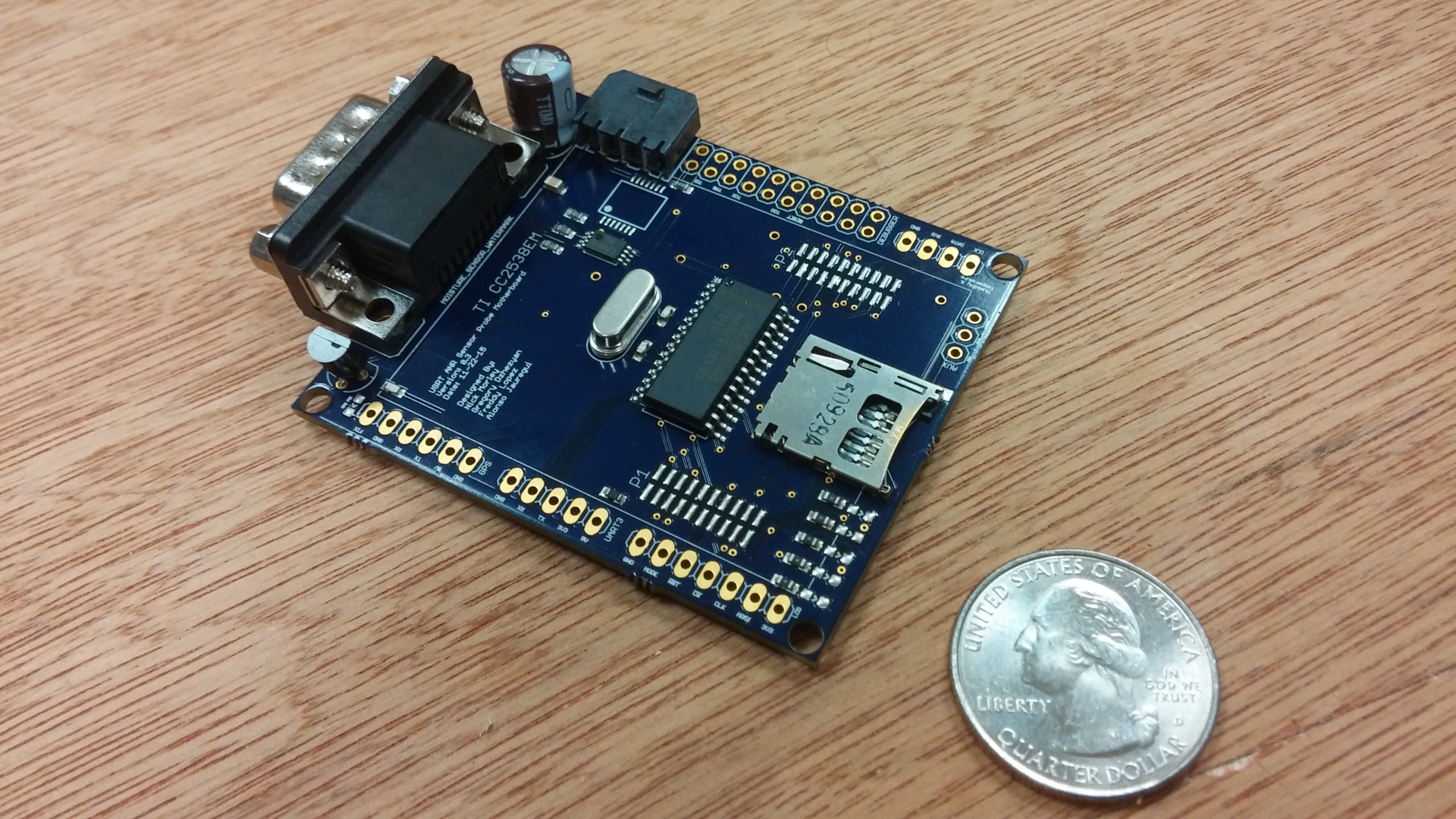
Potential for UAS Technology
This combined reduction of power, cost and size has provided a unique opportunity to provide cost-effective solutions for farm operations to employ smart technology with fields containing significant variability in moisture content, salinity, pH, etc. to optimize the application of fertilizer, water and pesticides for precision farming.
Under Dr. Kriehn, Fresno State University has been investigating the advantages of wireless remote sensing in conjunction with UAS technology to transmit data from soil probes and other sensors in the field for automated data collection that is correlated against spatial GPS coordinates in the field and orthomosaic images. Between 2013-2019, Fresno State developed a low-power, embedded sensor platform that integrated temperature, humidity and moisture sensors with a Texas Instruments microcontroller to create an automated data collection system for almond fields. The embedded platform stored sensor data on an integrated SD card until wirelessly transferring the data to a small UAS platform via a 2.4 GHz wireless radio.
A MEMS sensor was utilized to collect temperature and humidity data, and a Watermark 900 Soil Moisture monitor and sensors were used to collect soil moisture data which was then communicated to the microcontroller using standard electronic communication protocols. A GPS receiver was also used to date, time and location-stamp the data. After data was collected at specific (and re-configurable) time intervals, the microcontroller was placed in a sleep state to minimize energy consumption of the sensor platform. When data was ready to be transferred to the UAS drone, the microcontroller woke from the sleep state, read data from the SD card, and transmitted the information to the UAS platform using the wireless radio.
Additionally, Fresno State developed and refined necessary networking and communication protocols to ensure the ground sensor nodes could communicate effectively with the central “mother” node on the UAS platform for smoother automated data collection. Multiple field tests were conducted to test and validate the system, which lead to refining software protocols including GPS and a real-time clock for long-term data synchronization. Statistical delays were also added to the ground sensor nodes to avoid data collisions or data loss when multiple nodes received a wake-up chirp for data transfer and attempted to communicate with the UAS simultaneously.
The first field prototypes were successfully demonstrated during educational drone field days at farms in Riverdale, Calif. and near Los Banos, Calif. under the support of a UC-ANR grant in conjunction with UC Merced and the UC-ANR Cooperative Extension.
It is therefore anticipated that the continued integration of embedded sensor technology, advanced multi-spectral imagers, autonomous flight and data collection, next-generation UAS platforms with beyond line-of-sight navigation and collision avoidance systems, and sophisticated data analysis tools will increasingly allow farmers to optimize their yields while allowing for sustainable farming practices due to increased pressure on the world’s natural resources.
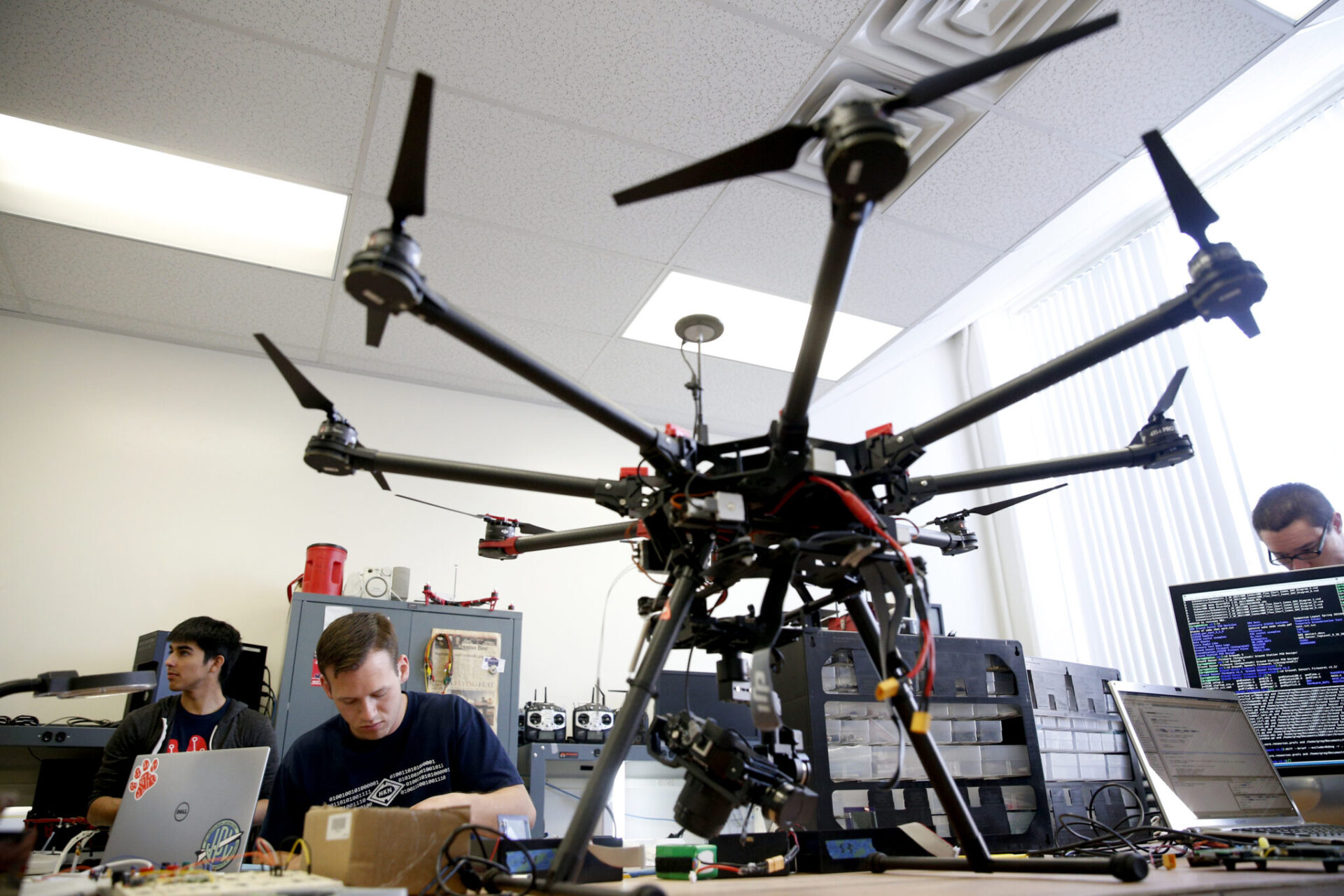